Use Case - Building a Retrieval Augmented Generation (RAG) Pipeline (featuring LlamaIndex)
Intro #
GenAI Studio simplifies the creation of Retrieval-Augmented Generation (RAG) applications. In this example, we’ll demonstrate how to set up a RAG pipeline using LLamaIndex with a GitHub dataset. This will help answer queries and generate responses in the GenAI playground.
Creating a Notebook #
First, we’ll create a notebook to work in. For detailed steps, visit creating a notebook.
Configuring the GenAI Studio Client #
Next, we import the necessary modules and set up the GenAI Studio client along with the configuration for our RAG pipeline. Here’s a sample of what that might look like:
from rag_pipeline.main import (
Config,
create_lore_client,
get_default_rag_pipeline_config,
get_default_rag_pipeline_run_config,
)
# Create a configuration object for your RAG pipeline
config = Config()
# Create a GenAI studio client
lore = create_lore_client()
Preparing the Vector Store #
Using GenAI Studio’s helper functions, we can create a vector store of dataset embeddings. In this example, we’re using ChromaDB but you can use any vector store or database:
from lore.integrations.helpers.rag import (
get_chromadb_client,
get_chromadb_collection,
get_chromadb_vector_store,
download_file,
insert_csv_to_chromadb,
)
# URL of the CSV file containing FAQs
COVID_FAQ_CSV_URL = "https://raw.githubusercontent.com/sunlab-osu/covid-faq/main/data/FAQ_Bank.csv"
# Create an embedding model
embed_model = create_embedding_from_rag_run_config(lore, run_config, "default")
# Set up the ChromaDB client and collection
chroma_client = get_chromadb_client(config.chroma_db_dir)
chroma_collection = get_chromadb_collection(chroma_client)
vector_store = get_chromadb_vector_store(chroma_collection)
# Download the CSV file and insert it into ChromaDB
csv_path = download_file(COVID_FAQ_CSV_URL, config.data_dir)
insert_csv_to_chromadb(vector_store, embed_model, csv_path, num_rows=3)
Creating the RAG Pipeline #
Finally, we create our RAG pipeline using the configuration and save it for use in the GenAI Studio playground.
from rag_pipeline.main import rt
from lore.types import enums as bte
pipeline_spec = rt.RagPipeline.from_dir(
SCRIPT_DIR / "rag_pipeline/",
name=config.pipeline_name,
entrypoint="main:ExampleRagPipeline",
framework=bte.RagFramework.LLAMA_INDEX,
default_config=get_default_rag_pipeline_config(config, lore),
pypi_dependencies=[*get_llama_index_requirements()],
)
# Save the RAG pipeline for use in the GenAI studio playground
pipeline_spec = lore.create_rag_pipeline(pipeline_spec)
Using the RAG Pipeline in the Playground #
With the RAG pipeline created, we can now hand it off to a subject matter expert (SME). The SME can easily interact with the pipeline and generate responses in the GenAI Studio playground.
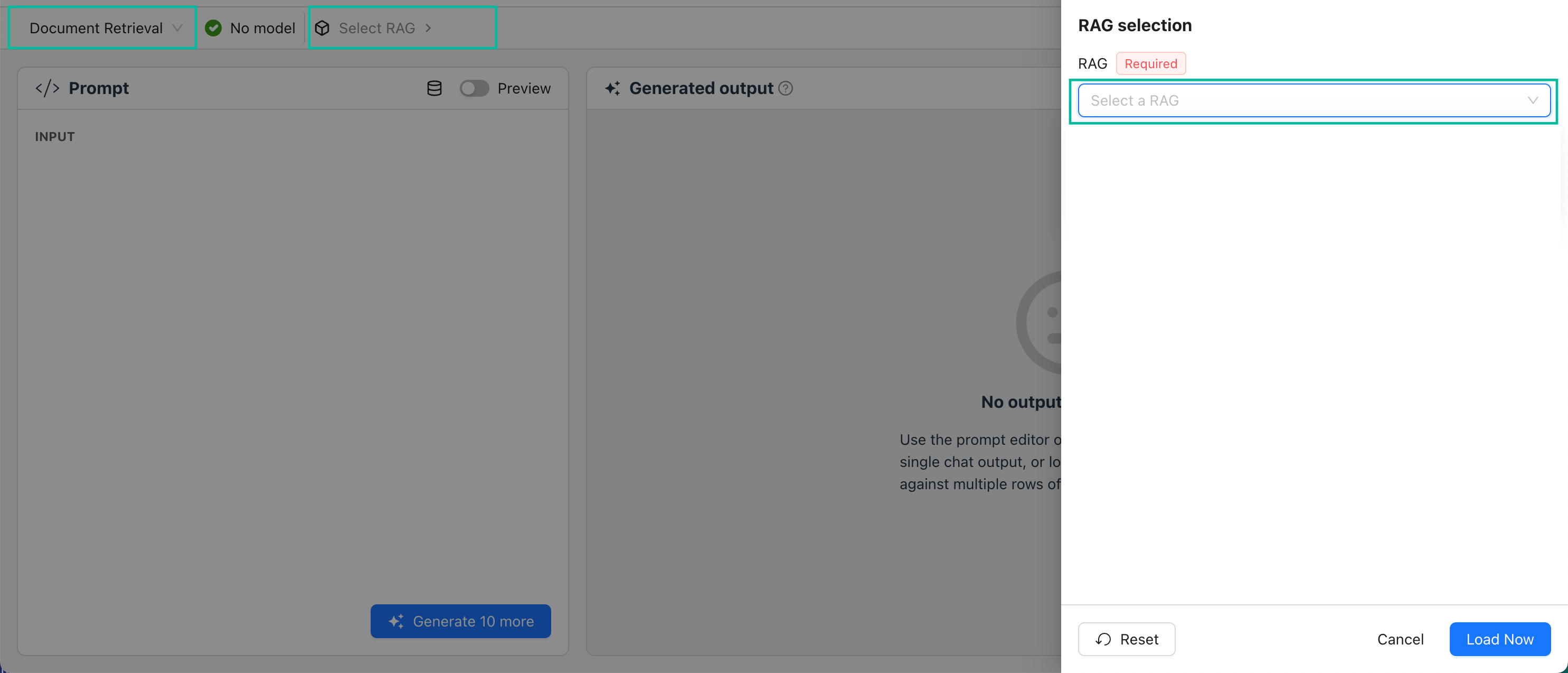